This doesn’t matter for purely predictive models though (assuming it’s cross-validated). SIERA is functioning strictly as a predictive model of park-adjusted ERA, so if they wanted to include a cubic term for the price of tea in China, more power to them if it works. The ensemble techniques that are best-in-class predictors produce the kind of uninterpretable ham sandwich mosaics that SIERA could only dream of. So my issue isn’t with the model being too fancy, having too many quadratics and interactions, or anything like that.
My issue is that they claim their only goal with SIERA was to beat the other models at predicting park-adjusted ERA, and then proceed to (badly) use a modeling technique (OLS regression) that is poorly-suited for that purpose while violating its assumptions and not providing any meaningful model diagnostics. This time it worked out, but think about how many amateurs hacking away at Excel end up building models that don’t work. We’re only seeing the lucky ones.
We have a pretty good idea about what the best prediction techniques are now, and it’s not what these guys are doing. So my complaint is that they’re (mis)using a hammer when a screwdriver is needed. Can you drive a screw using the sharp edge of the claw on a hammer? Maybe, but why would anyone do that? You’re familiar with the Netflix prize, right?
From one of the goat papers:
Each observation consisted of a user ID, a movie title, and the rating that the
user gave this movie. The task was to accurately predict the ratings of movie-user pairs for a test set such that the predictive accuracy improved upon Netflix’s
recommendation engine by at least 10%.
At the data exploration and reduction step, many teams including the winners found that the noninterpretable Singular Value Decomposition (SVD) data reduction method was key in producing accurate predictions: “It seems that models based on matrix factorization were found to be most accurate.” As for choice of variables, supplementing the Netflix data with information about the movie (such as actors, director) actually decreased accuracy:
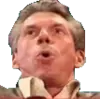
In terms of choice of methods, their solution was an ensemble of methods that included nearest-neighbor algorithms, regression models, and shrinkage methods. In particular, they found that “using increasingly complex models is only one way of improving accuracy. An apparently easier way to achieve better accuracy is by blending multiple simpler models.”
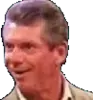
There are really no first principles or curve fittings or anything going on here. It’s just a computer throwing the kitchen sink at a large data set to see what sticks, and in ways that aren’t necessarily interpretable. The actual model it comes up with could be some crazy thing that far exceeds the complexity of a quadratic OLS with interactions–in fact, I’m certain it would be if there was a meaningful way to compile the ensemble contributors into a single interpretable thing. Gimme that all day over amateurs hacking around in Excel until they hit something.